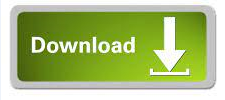
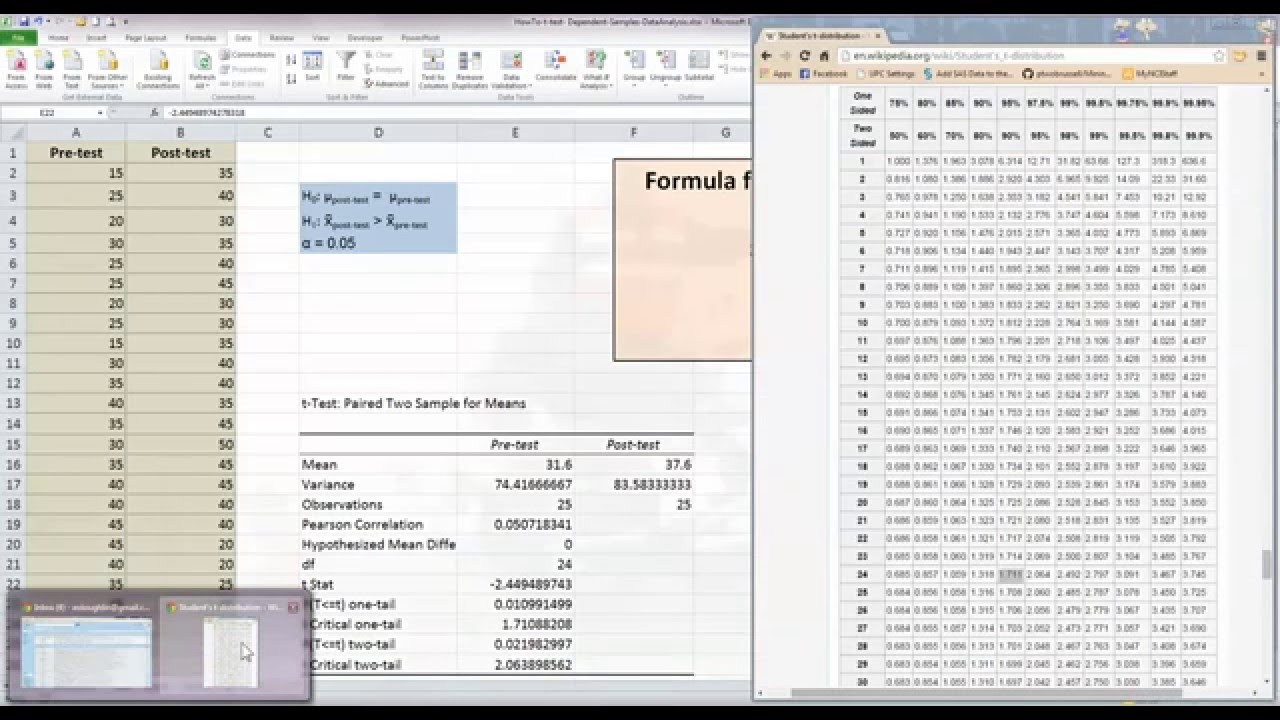
This value is smaller than any reasonable significance level. The E-12 indicates that we need to move the decimal point 12 places to the left. It’s written in scientific notation because it is a tiny value. Our p-value for the overall F-test is 8.93783E-12. If this test result is statistically significant, it suggests you have a good model. This test determines whether your model with all of its independent variables does a better job explaining the dependent variable’s variability than a model with no independent variables.
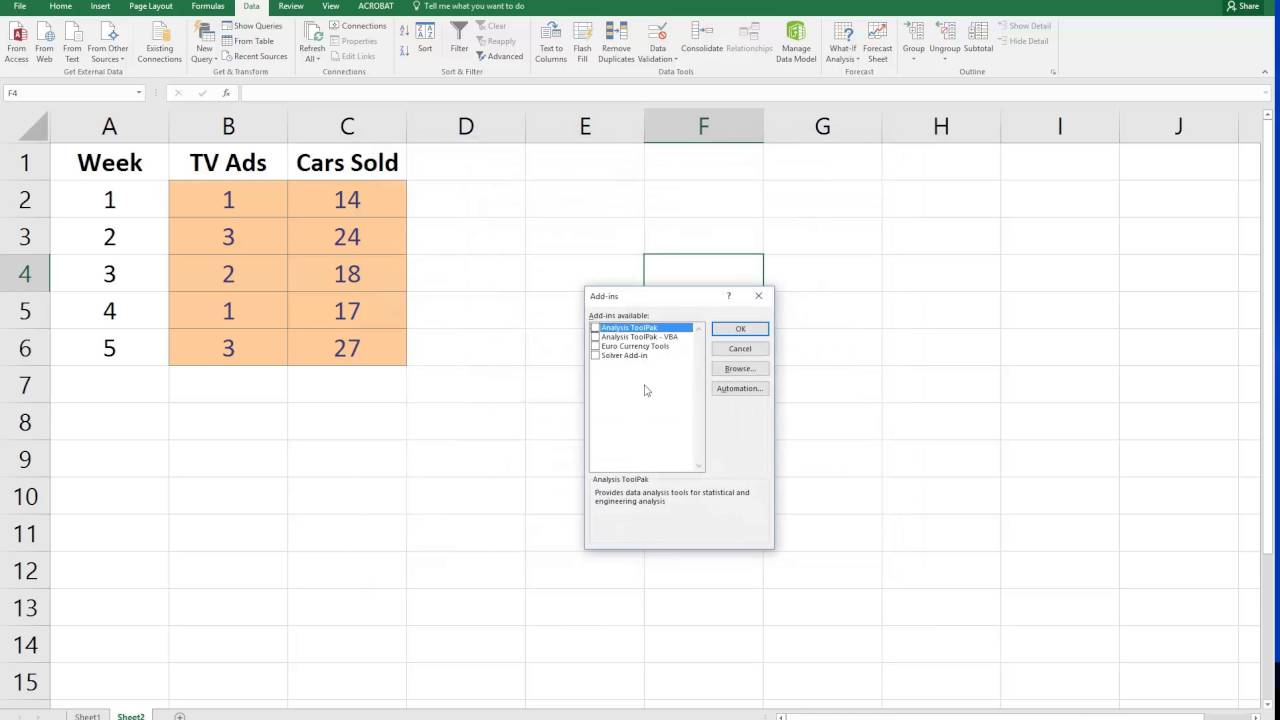
This is the p-value for the F-test of overall significance. In Excel’s ANOVA table, the most important statistic is Significance F. Conveniently, this value uses the measurement units of the dependent variable.įrom the output, we know that the standard distance between the predicted and observed values is 8.93 degrees Celsius.įor more information, read my posts about: You want lower values because it signifies that the distances between the data points and the fitted values are smaller. This statistic shows how wrong the regression model is on average. The standard error of the regression indicates the typical size of the residuals. For example, if you compare a model with one independent variable to a model with two, you often favor the model with the higher adjusted R-squared. The adjusted R-squared value helps us compare regression models with differing numbers of independent variables. However, there are important caveats about that! Usually, higher R-squared values are better.
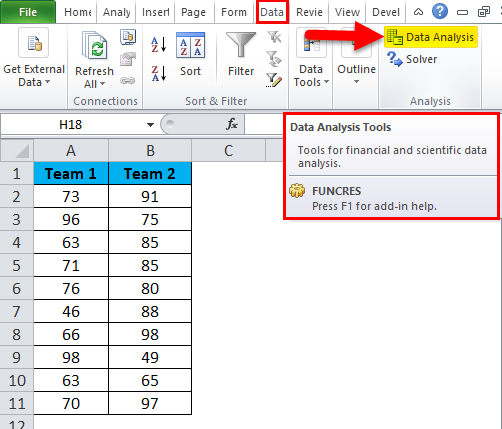
The R-squared value of ~0.858 indicates that our model accounts for about 85.8% of the dependent variable’s variance. So, we’ll skip it and go to the two R-squared values. Multiple R is not a standard measure for regression and it is difficult to interpret. The Regression Statistics table provides statistical measures of how well the model fits the data. If you want to learn more about the statistics, be sure to click the links for more detailed information! Regression Statistics Table We’ll work our way down from the top of Excel’s regression analysis output. Interpreting Excel’s Regression Analysis ResultsĪfter Excel creates the statistical output, I autofit some of the columns for clarity. Check Residual Plots to display the values of the residuals and graph them.įor this example, your popup should look like the following:.Check Confidence Level if you want to display confidence intervals for the coefficient estimates.Do not check this box unless you’re absolutely sure you know what you’re doing! For more information, read my post about the regression constant. Check the Constant is Zero if you want to force the regression line through the origin.This option makes the output easier to interpret. Check the Labels checkbox if you have meaningful variable names in row 1.On graphs, analysts place independent variables on the horizontal X-axis. These variables are also known as predictor variables, input variables, and are commonly denoted using Xs. However, in observational studies, the values of the independent variables are not set by researchers but observed instead. In randomized controlled experiments, researchers systematically set and change the values of the independent variables. Independent variables are the variables you include in the model to explain or predict changes in the dependent variable. In Excel, these variables must be next to each other so you can choose them all in one range. Under Input X Range, select the range for your independent variable(s).Traditionally, analysts graph dependent variables on the vertical Y-axis. It’s also known as the response variable, outcome variable, and it is commonly denoted using a Y. The values of this variable depend on other variables. The dependent variable is a variable that you want to explain or predict using the model. Under Input Y Range, select the range for your dependent variable.Step-by-Step Instructions for Filling In Excel’s Regression Box For more details about this process, read my post about Specifying the Correct Regression Model. Specifying the correct model is an iterative process where you fit a model, check the results, and possibly modify it.
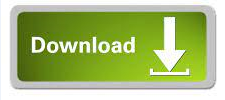